ARTIFICIAL
INTELLIGENCE
Plant electrophysiology signals encode information that plants use to regulate growth, development and defense. To build diagnostic algorithms plants are recorded in both healthy and stressed states.
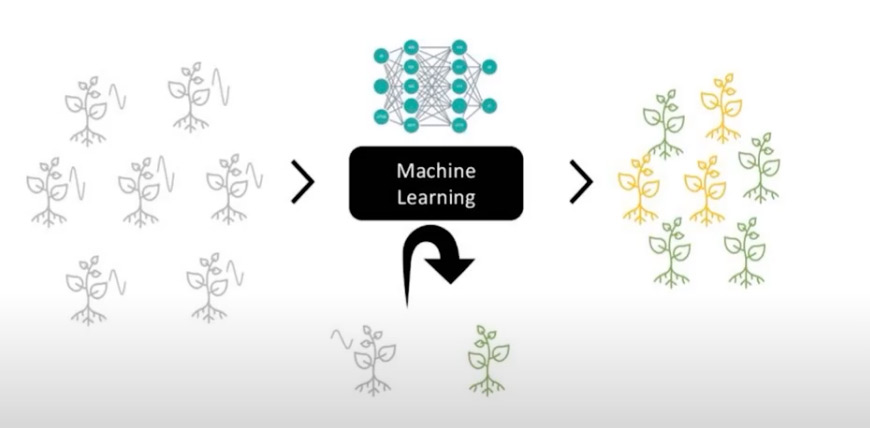
Recorded plant signals are then analysed using a range of statistical and machine learning methods to create models that detect and predict plant health states. Once a model is ready it can be deployed on the sensor and a real-time alert is generated to notify when the stressed state occurs.
Using our data base of over 150,000 days of recorded and annotated plant activity, we can estimate normal behavior in many different growing conditions for a variety of crops, we can calibrate which deviances from normal behavior require immediate attention, and even predict their impact on yield’s quantity and quality. We can work with any type of plant.
To calibrate the predictions to the individual client’s environment, it’s sufficient to acquire a few days of data from severalneighboring plants. For additional accuracy we annotate the data with information such as the substrate composition, the irrigation type, or the plant life stage at the beginning of the recording.
Our family of digital solutions hinges on classification and regression models as well as statistical inference. Examples include predictive models based on fully connected Deep Neural Network (DNN) or Long-Short Term Memory models (LSTM), and state classifiers based on Hidden Markov Models (HMM) or k-Nearest Neighbors (KNN).